Design Optimization Using Value Equations
Quality Function Deployment (QFD) is an excellent tool to ensure linkage of customer needs to product requirements. This article will provide a high-level overview on creating a ‘first-level’ QFD and how it can be used to guide design optimization.
(There are many additional features of QFD , however, and readers of this article are encouraged to research the methodology further.)
As stated above, we use the QFD matrix (similar to a cause-effect matrix) to ensure linkage of customer needs to product requirements using the critical thinking / questions as follows:
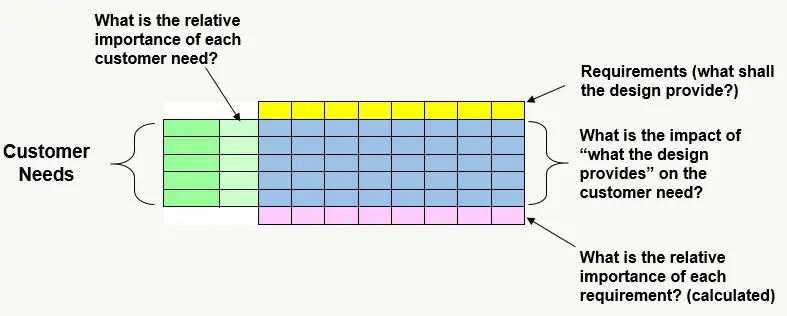
Let’s dive right into a generic example of a filled-out QFD matrix:
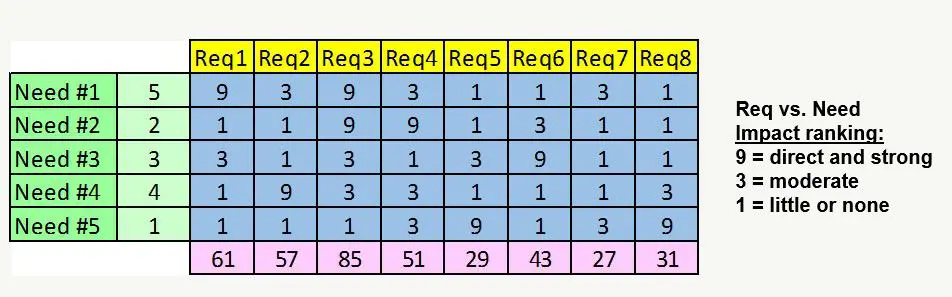
This QFD suggests a value equation as follows:
Customer Value = 8.5*Req3 + 6.1*Req1 + 5.7*Req2 + 5.1*Req4 + 4.3*Req6….etc
How can we use equation? It depends on the requirement:
- If ‘more is better’…for example Req3 performance can be increased, then this increases overall value to the customer with the multiplier 8.5. Improving Req6 won’t add as much value (according to this analysis).
- If less is better, then we would replace a “+” sign with “-” sign in the equation.
- Moving upper and lower specification limits closer together would also increase value assuming less variability is desireable (a more complex equation would be required to reflect this).
- In the equation above, customer value is simply an index, however value can be converted to dollars (especially when compared to a competitive product). We would then be able to assign a dollar value for improved performance or reduced variability, thereby tying product performance to pricing. The value of adding a capability could also be estimated.
- The Critical-to-Quality characteristics (CTQs) are Req3, Req1 and Req2. All requirements must be met, however, we would want to focus our product development efforts on ensuring those are optimized first and foremost since they have the biggest impact on customer needs.
- Not all requirements represent a variable performance parameter….in other words a desired functional output of a product might be binary (either it exists or it doesn’t). If it provides value it should still be represented in the equation, however, perhaps as a constant.
Rather than subjective judgment, use mathematics to support design optimization decisionmaking
Your equation may not be perfect, but we at least have a mathematical relationship by which value can be estimated and tied to product performance and features. Also, our goal is to minimize product cost: an index CV/C could be maximized through the combination of a value equation and cost models (a design optimization model which enables trade-off decisions to be estimated for maximized value and minimized cost).
This article only scratches the surface on the topic…hopefully it provides the reader enough information on the concept of value equations and using mathematical relationships to enable optimized designs.